Based on our day to day experience, the information we consume is entirely digital. We read the news on our mobile devices far more than we do from printed copy newspapers. Tickets for sporting events, music concerts, and airline travel are stored in apps on our phones. One could go weeks or longer without needing to have any paper currency in his or her wallet, as digital payments are ubiquitous. However, many companies across different industries still primarily operate on manual, paper-based processes. For example, healthcare payors, construction companies, and law firms deal with billions of documents and forms, making the process of finding information difficult and time-consuming. When documents are found, extracting information through manual data entry can be slow, expensive, and error prone, resulting in increases in compliance risks. Furthermore, domain experts need to identify and categorize domain-specific phrases and keywords (or entities), or use traditional Optical Character Recognition (OCR) and keyword detection software that requires manual customization. These approaches can create scrambled output and unusable results. AWS AI services such as Amazon Kendra, Amazon Textract, Amazon Comprehend, and Amazon Comprehend Medical help solve these challenges by automating data extraction and comprehension using machine learning (ML).
Overview of the Document Understanding Solution
The Document Understanding Solution (DUS) allows you to use the power of AWS AI for enterprise search, document digitization, discovery, and extraction and redaction of select information. Part of the Intelligent Document Processing services offered from AWS, this solution uses AWS artificial intelligence (AI) services to solve business problems.
Search and discovery
These challenges exist in almost every business vertical. Imagine a manufacturer that has to maintain archives of thousands – if not millions – of product and tool specifications. Without document digitization of archives, there could be massive underutilization of their highly valuable tool data and information retrieval could be complex and costly. In another example, a company in the financial industry could have 1000s of financial reports in paper format. Without a simple way to extract and digitize this data, it could take an extensive manual effort to keypunch it.
To help with these situations, DUS leverages multiple ML services, including Amazon Textract. Amazon Textract is a fully managed machine learning service that automatically extracts text and data from scanned documents that goes beyond simple optical character recognition (OCR) to identify, understand, and extract data from forms and tables. Amazon Textract will move the data from the documents to a format that can be readily searched. Next, Amazon Kendra and Amazon Elasticsearch Service (Amazon ES) are available to provide the end user search experience in DUS. Amazon Kendra is an intelligent search service powered by machine learning. Amazon Kendra uses ML to obtain better results for natural language questions, and will return an exact answer from within a document, whether that is a text snippet, FAQ, or a PDF document. In addition to Amazon Kendra, the DUS provides a rich search experience to the user through the use of Amazon Elasticsearch Service. Amazon Elasticsearch Service is a fully managed service that makes it easy for you to deploy, secure, and run Elasticsearch cost effectively at scale.
Control and compliance
In addition to search, the ability to analyze documents at scale is essential. Amazon Textract extracts text from documents, which can then be input into Amazon Comprehend or Amazon Comprehend Medical. Amazon Comprehend is a natural language processing (NLP) service that uses machine learning to find insights and relationships in text. It can identify key phrases and entities, such as places, people, and brands. Amazon Comprehend Medical is similar to Comprehend. It is a natural language processing service that makes it easy to use machine learning to extract relevant medical information from unstructured text. It can identify medical entities, such as medical conditions and medications.
Identifying these key pieces of information allows for compliance controls through redaction. For example, an insurer could use this solution to feed a workflow that automatically redacts personally identifiable information (PII) or protected health information (PHI) for their review before archiving claim forms by automatically recognizing the important key-value pairs and entities that require protection.
Other industries can also use this solution for complying with regulatory standards, such as GDPR and HIPAA. For example, this solution could be used by a law firm to redact PII, organization names or brand names. Another example includes a security agency needing to redact all vital information such as names, locations and/or dates from a case file for data security or privacy concerns.
Workflow automation
The DUS solution delivers results at scale in production workflows. Organizations can more rapidly process documents such as insurance claims and forms, and seamlessly extract tables from PDFs into CSVs to conduct additional analysis. With detection and categorization of medical entities and ICD-10-CM ontologies, medical institutes can recognize exponential savings in workforce, time, and other resources that are spent identifying and classifying patient information. All the data is stored by the solution in easily accessible formats, such as CSV and JSON files, which can be fed into downstream pipelines. Additionally, the bulk processing feature in DUS allows you to import a large number of documents directly for processing and analysis.
The following diagram illustrates the DUS architecture.
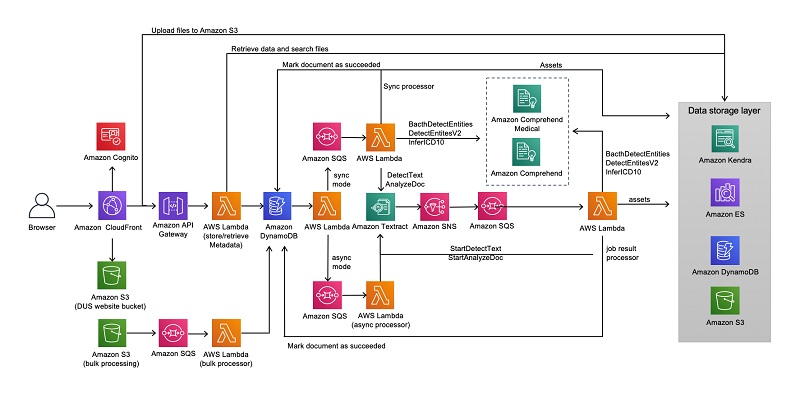
Deploying DUS
For instructions on setting up DUS, see Document Understanding Solution on AWS Solutions.
Deploying DUS sets up a web application that you can use for document understanding. The deployment includes setting up infrastructure in your AWS account and pre-loading sample documents.
Using DUS
Once you have successfully completed deploying the DUS demo, you are then provided with instructions on how to login into the application. After logging in, you are directed to the homepage, as seen below. You have three options which cover the common use-cases in document understanding solution: Discovery, Compliance, and Workflow Automation.
When you select the Discovery track you will be directed to the preloaded documents page or the Document List page. You may select one of the preloaded sample documents or upload your own document. From here, you can search for a specific document by using a phrase or keyword.
If you decide to upload your own document, choose upload your own documents above the available documents. You will then be directed to a new page to upload your own documents. This page also has sample documents from different industry verticals for you to experiment with.
Back on the Document List page, you will find some PDF and image files. Text in these documents are not actually tagged or available to use by default. However, since these documents have been processed by the solution, you will now be able to search for information within these documents. If you decide to search for a specific phrase or keyword in the search bar, then the solution will analyze the text it has extracted from the documents and provide you with search results. The search results can be displayed in three different ways; a comparative view of Amazon ES (traditional search) and Amazon Kendra (semantic search), just Amazon ES or just Amazon Kendra .
For Amazon Kendra results, you also have the option to provide feedback by either up-voting or down-voting an Amazon Kendra suggested answer.
Amazon Kendra also supports filtering based on user context. Under the Amazon Kendra results view, you can filter results based on the users for the preloaded documents. Click the Filter button to the right of the Amazon Kendra Results title. You can then select a persona and one of the suggested questions to display filtered results. Amazon Kendra will then rank results based on the selected persona. You can toggle between the various personas to compare how the results differ. For demonstration purposes, the Document Understanding Solution comes with preloaded documents and personas from the medical industry. You will be able to notice that based on the question and persona selected, results are ranked differently creating a more targeted search experience for the user.
From the Document List search results view, you can select a document that you want to further explore. This will direct you to the Document Details page. See the following image.
The following image shows the tool bar above the search bar, where you can choose to see different types of information from the document.
The tabs have the following functions:
- Preview – Under this tab, you are able to view the original document as well as download a searchable PDF version of the document. This helps users to convert their documents – be it images or PDFs into easily searchable PDF files.
- Raw Text – Under this tab, you can access all the text identified in the file.
- Key-Value Pairs – Under this tab, key-value pairs from the document are highlighted. In this process, all forms in the document are identified and stored in a key-value pair format. If desired, you can download a CSV file of the key-value pairs. This is especially useful for organizations that have structured data and would want to automate their data extraction and storage workflows. For example, organizations that have a lot of forms like job applications or medical patient forms.
- Tables – Under this tab, you can view all the tables identified in the document. Like the key-value pairs, you can download the tables in the CSV format. Companies dealing with balance sheets or with invoices would find this feature extremely useful since it allows users to easily convert tables, images and PDFs into CSV files which can then be used for further analysis.
- Entities and Medical Entities – Under these tabs, you can find the general and medical entities in the document respectively. These entities include persons, locations, dates, PHI and medical information which helps organization to easily identify and extract critical medical data in a document.
For exploring redaction controls, choose the Compliance option on the toolbar. Here you can choose to redact information like key-value pairs, entities, medical entities or even keyword matches by switching to the respective tabs on the tool bar and choosing Redact. One example of how this feature may be useful is to consider a clinic that wants to redact PHI information before they decide to share medical records. Another example is an organization that wants to redact specific information identified as keylue pairs in forms present in their documents. As seen in the following image, you can redact information, download the redacted document and even clear redactions after use.
In terms of Workflow Automation, the Document Understanding Solution also provides some input and output capabilities via the AWS Console which makes it easier to integrate DUS into an existing pipeline. DUS supports a bulk document processing mode, in which you can simply input documents into an Amazon Simple Storage Service (Amazon S3) bucket which will be asynchronously analyzed and made available in the application. More information on bulk processing is available on the AWS Solutions Implementation Guide. Results from the different AWS AI services are all stored within Amazon S3 buckets and the corresponding metadata is available in Amazon DynamoDB tables. This helps users of the solution to build downstream pipelines from these datastores that hold the document analysis data.
Summary:
This post reviewed how you can integrate Amazon Textract, Amazon Comprehend, Amazon Comprehend Medical, and Amazon Kendra to conduct enterprise search, document digitization, document discovery, and extraction and redaction of select information.
To access the DUS source code, see Document Understanding Solution on GitHub. This solution has been made open source so that you can extend and incorporate the solution into your AWS workflows.
About the Authors
Simran Baxendale is a Program Manager in the Amazon Machine Learning Solutions Lab. She helps define, coordinate and execute program strategy for the demos applications team.
Curtis Bray is a manager in the Amazon Machine Learning Solutions Lab. He leads the demos applications team that focuses on building use case based demos that show customers how to unlock the power of AWS AI/ML services to solve real world business problems.
Alex Chirayath is an SDE in the Amazon Machine Learning Solutions Lab. He helps customers adopt AWS AI services by building solutions to address common business problems.
from AWS Machine Learning Blog https://ift.tt/3oHLhue
via A.I .Kung Fu